Previous Projects
In the following, an overview of previous research projects within the group is provided.
User feedback is essential for the control of personalized devices to ensure user satisfaction. In a user feedback control solution, the user provides feedback to the controller analogously to sensors.
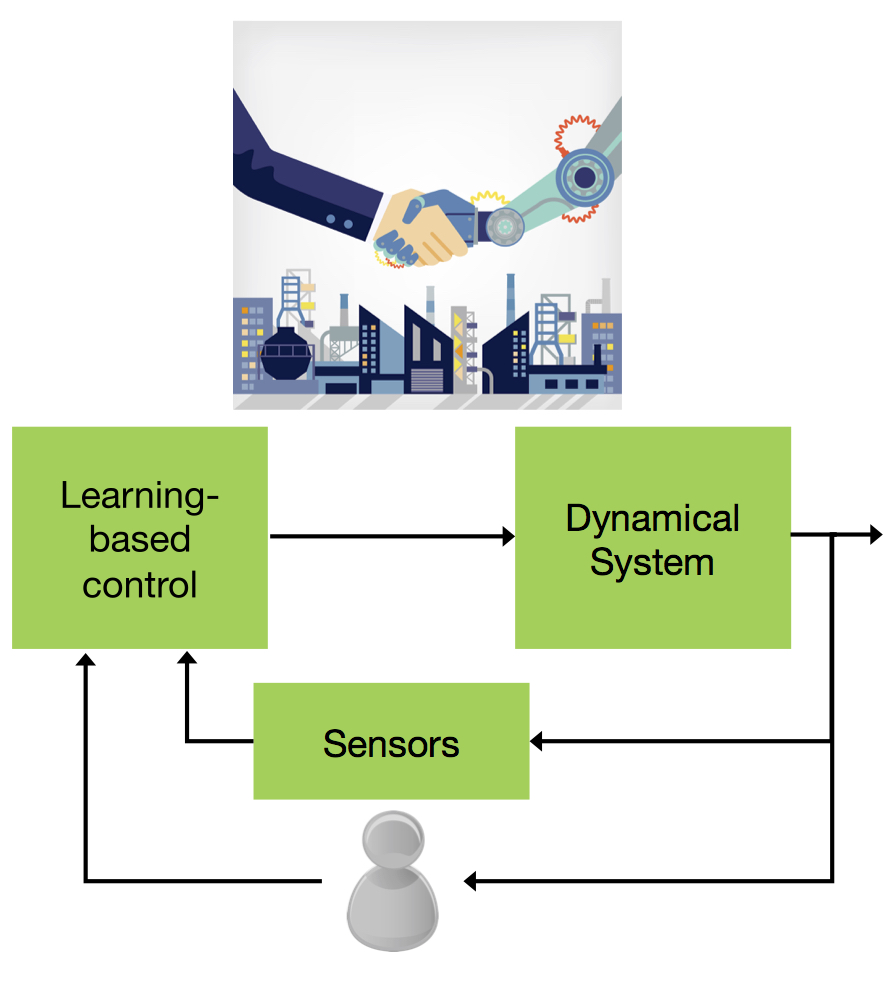
We model and learn users' demands with two approaches: Firstly, we learn a priori unknown user-specific state transition probabilities in a Markov model with restless multi-armed bandit experiments, where the state transition probabilities originate from discrete controller decisions and the states correspond to the user’s satisfaction level. Secondly, a user-specific cost or reward function is predicted in an inverse optimal control framework.
Selected Publications
Marcel Menner, Lukas Neuner, Lars Lünenburger, and Melanie N. Zeilinger. external page Using Human Ratings for Feedback Control: A Supervised Learning Approach with Application to Rehabilitation Robotics. IEEE Transactions on Robotics, 2020.
Marcel Menner, Peter Worsnop, and Melanie N. Zeilinger. external page Constrained Inverse Optimal Control with Application to a Human Manipulation Task. IEE Transaction on Control Systems Technology, 2021.
Marcel Menner and Melanie N. Zeilinger. external page A User Comfort Model and Index Policy for Personalizing Discrete Controller Decisions. Proceedings of the European Control Conference, Limassol, Cyprus, 2018.
Marcel Menner and Melanie N. Zeilinger. external page Convex Formulations and Algebraic Solutions for Linear Quadratic Inverse Optimal Control Problems. Proceedings of the European Control Conference, Limassol, Cyprus, 2018.
Contact Persons
Marcel Menner (MERL)
Funding Sources
This work was supported by the Swiss National Science Foundation under grant no. PP00P2_157601/1.
While traditionally, power supply matches the fixed demand at any given time, modernized grids can leverage the flexibility of demand for utilizing resources more efficiently, often referred to as demand-side management. In particular electric vehicles represent not only a large load, but offer significant flexibility and the compelling capability of storing and providing energy for the grid. In addition, many traditional household loads can be deferred depending on the grid load. For example, a costumer may want the vehicle to be charged or dishes to be washed by a certain time, regardless of when the power is provided. This represents an enormous new potential for balancing a grid that relies on highly uncertain renewable energy.
We focus on three main challenges in this problem: 1) Load connections vary due to their utilization by the costumer, who is generally not willing to compromise on the connection times; 2) Grid safety has to be guaranteed, e.g. lines cannot be overloaded; 3) The optimization of large populations of distributed loads has to be performed efficiently.
We have developed a new approach for optimizing load profiles to reduce peak power in a distribution grid that can deal with varying load connections on the fly in a collaboration with UC Berkeley. More details can be found in the references below.

Selected Publications
S. Bansal, M. N. Zeilinger and C. J. Tomlin. external page Plug-and-play model predictive control for electric vehicle charging and voltage control in smart grids. Proc. IEEE Conf. on Decision and Control, 2016, pages 5894-5900.
C. Le Floch, S. Bansal, C. J. Tomlin, S. Moura and M. N. Zeilinger. external page Plug-and-play model predictive control for load shaping and voltage control in smart grids. IEEE Transactions on Smart Grid, 2017.
M. Zellner, T. T. De Rubira, G. Hug, and M. N. Zeilinger. external page Distributed Differentially Private Model Predictive Control for Energy Storage. IFAC World Congress, 2017.
Funding Sources
This work was supported by the Swiss National Science Foundation under grant no. PP00P2_157601/1.