Invited Session Series on Learning-based Control
Organizers:
external page Angela P. Schoellig (University of Toronto, Canada)
external page Sebastian Trimpe (RWTH Aachen University, Germany)
Melanie N. Zeilinger (ETH Zürich, Switzerland)
external page Matthias Müller (Leibniz University Hannover, Germany)
Data science and machine learning have demonstrated tremendous success in the last decades in applications such as image recognition, recommender systems, or question answering. Compared to these applications, which can largely be subsumed as static problems, learning-based control systems take a special role within the world of statistical and machine learning. The coupling of a learning algorithm with a control loop requires a combined treatment as a dynamic process and raises fundamental questions about stability, robustness, and safety, which are generally less critical in most traditional application areas of machine learning. In order to leverage the potential of data-based and learning methods for control, we therefore believe that principled approaches integrating machine learning and control theory are needed, which extend beyond the methods and tools of the individual disciplines.
Based on the increasing interest in this domain, we have started a new Invited Session Series on Learning-based Control taking place yearly at the IEEE Conference on Decision and Control (CDC). The sessions in 2016 through 2023 were a great success and we are looking forward to your contributions and the next session at CDC'24. Please do not hesitate to contact us if you have any questions.
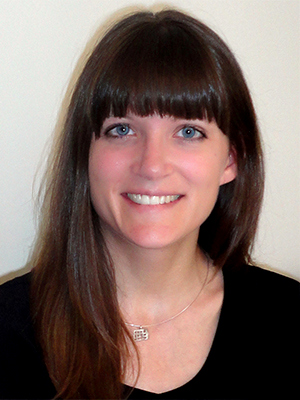
Angela Schoellig,
Angela Schoellig is an Assistant Professor at the University of Toronto Institute for Aerospace Studies (UTIAS), where she heads the Dynamic Systems Lab. She is also an Associate Director of the Center for Aerial Robotics Research and Education (CARRE) at the University of Toronto. With her team, she conducts research at the interface of robotics, controls and machine learning. Her goal is to enhance the performance and autonomy of robots by enabling them to learn from past experiments and from each other.
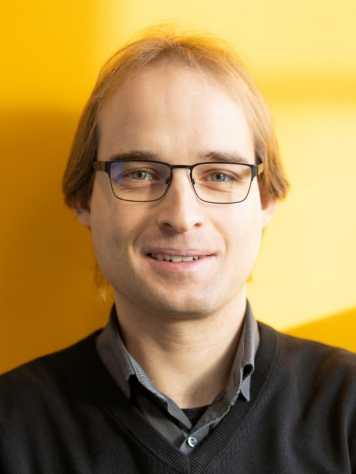
Sebastian Trimpe, external page
Sebastian is a Full Professor and Director of the Institute for Data Science in Mechanical Engineering (DSME) at RWTH Aachen University, Germany. DSME focusses on fundamental research at the intersection of control, machine learning, data science, and distributed systems, as well as innovative applications in cyber-physical systems, robotics, and industrycollaborations.
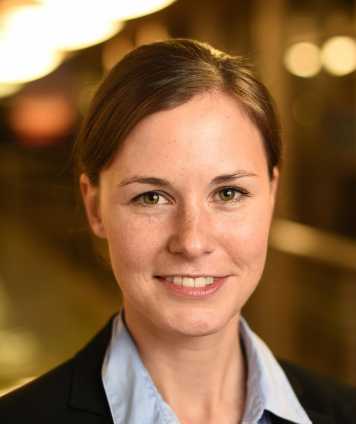
Melanie Zeilinger,
Melanie is an Assistant Professor at the Department of Mechanical and Process Engineering at ETH Zurich, where she is leading the Intelligent Control Systems group at the Institute for Dynamic Systems and Control. Her research interests are centered around real-time and distributed control and optimization, as well as safe learning-based control, with applications to human-in-the-loop control.
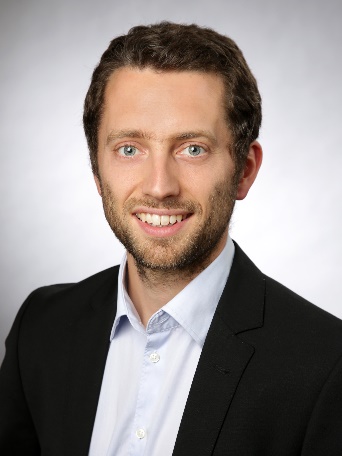
Matthias Müller,
Matthias is Full Professor and Director of the Institute of Automatic Control at the Leibniz University Hannover, Germany. His research interests include nonlinear control and estimation, model predictive control, data-based and distributed control, with application in different fields including biomedical engineering.
This Invited Session is supported by the external page Max Planck ETH Center for Learning Systems and the external page IEEE CSS Technical Committee on Intelligent Control.
The Invited Session was very well received among CDC participants. We thank all authors for contributing to this success.
Session overview:
- external page Distributed Iterative Learning Control for a Team of Quadrotors
Hock, Andreas (Univ. of Toronto)
Schoellig, Angela P (Univ. of Toronto) - external page Continuous-Time DC Kernel — a Stable Generalized First Order Spline Kernel
Chen, Tianshi (Linköping Univ. Sweden)
Pillonetto, Gianluigi (Univ. of Padova)
Chiuso, Alessandro (Univ. Di Padova)
Ljung, Lennart (Linkoping Univ) - external page Learning Quadrotor Dynamics Using Neural Network for Flight Control
Bansal, Somil (UC Berkeley)
Akametalu, Anayo K. (UC Berkeley)
Tomlin, Claire J. (UC Berkeley)
Laine, Forrest J. (UC Berkeley) - external page Safe Learning of Regions of Attraction for Uncertain, Nonlinear Systems with Gaussian Processes
Berkenkamp, Felix (ETH Zurich)
Moriconi, Riccardo (ETH Zurich)
Schoellig, Angela P (Univ. of Toronto)
Krause, Andreas (ETH Zurich) - external page Learning State Representation for Deep Actor-Critic Control
Munk, Jelle (Delft Univ. of Tech)
Kober, Jens (Delft Univ. of Tech)
Babuska, R. (Delft Univ. of Tech) - external page Relaxation of the EM Algorithm Via Quantum Annealing for Gaussian Mixture Models
Miyahara, Hideyuki (The Univ. of Tokyo)
Tsumura, Koji (The Univ. of Tokyo)
Sughiyama, Yuki (The Univ. of Tokyo)
The Invited Session was very well received among CDC participants. We thank all authors for contributing to this success.
Session overview:
- external page Goal-Driven Dynamics Learning Via Bayesian Optimization
Bansal, Somil (UC Berkeley)
Calandra, Roberto (UC Berkeley)
Xiao, Ted (Machine Learning at Berkeley)
Levine, Sergey (UC Berkeley)
Tomlin, Claire J. (UC Berkeley) - external page Data-Driven Approximate Dynamic Programming: A Linear Programming Approach
Sutter, Tobias (ETH Zurich)
Kamoutsi, Angeliki (ETH Zurich)
Mohajerin Esfahani, Peyman (TU Delft)
Lygeros, John (ETH Zurich)
- external page Stable Gaussian Process Based Tracking Control of Lagrangian Systems
Beckers, Thomas (Tech. Univ. of Munich)
Umlauft, Jonas (Tech. Univ. of Munich)
Kulic, Dana (Univ. of Waterloo)
Hirche, Sandra (Tech. Univ. München) - external page Trajectory Tracking and Iterative Learning on an Unmanned Aerial Vehicle Using Parametrized Model Predictive Control
Sferrazza, Carmelo (ETH Zurich)
Muehlebach, Michael (ETH Zurich)
D'Andrea, Raffaello (ETH Zurich)
- external page On the Design of LQR Kernels for Efficient Controller Learning
Marco Valle, Alonso (Max Planck Inst. for Intelligent Systems)
Hennig, Philipp (Max Planck Inst. for Intelligent Systems)
Schaal, Stefan (Univ. of Southern California)
Trimpe, Sebastian (Max Planck Inst. for Intelligent Systems) - external page Design of Deep Neural Networks As Add-On Blocks for Improving Impromptu Trajectory Tracking
Zhou, Siqi (Univ. of Toronto)
Helwa, Mohamed K. (Univ. of Toronto)
Schoellig, Angela P (Univ. of Toronto)
The Invited Session was very well received among CDC participants. We thank all authors for contributing to this success.
Session overview:
- external page Classification of Light Charged Particles Via Learning-Based System Identification (I)
Mazzoleni, Mirko (University of Bergamo)
Scandella, Matteo (University of Bergamo)
Formentin, Simone (Politecnico Di Milano)
Previdi, Fabio (Università Degli Studi Di Bergamo) - external page Learning-Based Model Predictive Control for Safe Exploration (I)
Koller, Torsten (Albert-Ludwigs-Universität Freiburg)
Berkenkamp, Felix (ETH Zurich)
Turchetta, Matteo (ETH Zurich)
Krause, Andreas (ETH Zurich) - external page Sample Complexity of Networked Control Systems Over Unknown Channels (I)
Gatsis, Konstantinos (University of Pennsylvania)
Pappas, George J. (University of Pennsylvania) - external page Efficient Encoding of Dynamical Systems through Local Approximations (I)
Solowjow, Friedrich (Max Planck Institute for Intelligent Systems)
Mehrjou, Arash (Max Planck Institute for Intelligent Systems)
Schölkopf, Bernhard (MPI for Biological Cybernetics)
Trimpe, Sebastian (Max Planck Institute for Intelligent Systems) - external page An Uncertainty-Based Control Lyapunov Approach for Control-Affine Systems Modeled by Gaussian Process
Umlauft, Jonas (Technical University of Munich)
Pöhler, Lukas (Technical University of Munich)
Hirche, Sandra (Technische Universität München) - external page Bayesian Nonparametrics and Feedback-Linearisation of Discretised Control-Affine Systems
Calliess, Jan-Peter (University of Oxford)
Roberts, Stephen (University of Oxford)
Papachristodoulou, Antonis (University of Oxford)
The Invited Sessions (WeA23, WeB23 and WeC23) were very well received by CDC participants. We thank all authors for contributing to this success.
Session Overviews:
WeA23 Invited Session: Learning-Based Controller Synthesis
- external page Deep Reinforcement Learning with Feedback-Based Exploration
Scholten, Jan Jelmer (Delft University of Technology)
Wout, Daan (Delft University of Technology)
Celemin, Carlos (Delft University of Technology)
Kober, Jens (Delft University of Technology) - external page Inverse Learning for Human-Adaptive Motion Planning
Menner, Marcel (ETH Zurich)
Berntorp, Karl (Mitsubishi Electric Research Labs)
Zeilinger, Melanie N. (ETH Zurich)
Di Cairano, Stefano (Mitsubishi Electric Research Labs) - external page A Data-Driven Policy Iteration Scheme Based on Linear Programming
Banjac, Goran (ETH Zurich)
Lygeros, John (ETH Zurich) - external page Bayesian Kernel-Based Linear Control Design
Schampicchio, Anna (University of Padova)
Chiuso, Alessandro (Univ di Padova)
Formentin, Simone (Politecnico di Milano)
Pillonetto, Gianluigi (University of Padova) - external page Closed-Loop Model Selection for Kernel-Based Models Using Bayesian Optimization
Beckers, Thomas (Technical University of Munich)
Bansal, Somil (UC Berkeley)
Tomlin, Claire J. (UC Berkeley)
Hirche, Sandra (Technische Universität München) - external page Learning Robust LQ-Controllers Using Application Oriented Exploration
Ferizbegovic, Mina (KTH Royal Institute of Technology)
Umenberger, Jack (Uppsala University)
Hjalmarsson, Hakan (KTH Royal Inst. Of Tech)
Schön, Thomas (Bo) (Uppsala University)
WeB23 Invited Session: Model Learning for Control
- external page An Efficient Reachability-Based Framework for Provably Safe Autonomous Navigation in Unknown Environments
Bajcsy, Andrea (UC Berkeley)
Bansal, Somil (UC Berkeley)
Bronstein, Eli (UC Berkeley)
Tolani, Varun (UC Berkeley)
Tomlin, Claire J. (UC Berkeley) - external page Local Asymptotic Stability Analysis and Region of Attraction Estimation with Gaussian Processes
Lederer, Armin (Technical University of Munich)
Hirche, Sandra (Technische Universität München) - external page Learning for Control: A Bayesian Scenario Approach
Garatti, Simone (Politecnico Di Milano)
Campi, M.C. (Universita’ Di Brescia) - external page One-Shot Verification of Dissipativity Properties from Input-Output Data
Romer, Anne (University of Stuttgart)
Berberich, Julian (University of Stuttgart)
Koehler, Johannes (University of Stuttgart)
Allgöwer, Frank (University of Stuttgart) - external page Active Training Trajectory Generation for Inverse Dynamics Model Learning with Deep Neural Networks
Zhou, Siqi (University of Toronto)
Schoellig, Angela P. (University of Toronto) - external page Hierarchical Event-Triggered learning for Cyclically Excited Systems with Application to Wireless Sensor Networks
Beuchert, Jonas (Technische Universität Berlin)
Solowjow, Friedrich (Max Planck Institute for Intelligent Systems)
Raisch, Joerg (Technical University, Berlin)
Trimpe, Sebastian (Max Planck Institute for Intelligent Systems)
Seel, Thomas (Technische Universität Berlin)
WeC23 Invited Session: Learning-Based Model Predictive Control - external page Regularized and Distributionally Robust Data-Enabled Predictive Control
Coulson, Jeremy (ETH Zurich)
Lygeros, John (ETH Zurich)
Dörfler, Florian (Swiss Federal Institute of Technology (ETH) Zurich) - external page Sample-Based Learning Model Predictive Control for Linear Uncertain Systems
Rosolia, Ugo (UC Berkeley)
Borrelli, Francesco (University of California at Berkeley) - external page Model Predictive Control Using Efficient Gaussian Processes for Unknown Disturbance Inputs
Grasshoff, Jan (Universität Zu Lübeck)
Maennel, Georg (Universität Zu Lübeck)
Abbas, Hossam (University of Lübeck)
Rostalski, Philipp (University of Luebeck) - external page Performance-Oriented Model Learning for Data-Driven MPC Design
Piga, Dario (University of Applied Sciences and Arts of Southern Switzerland)
Forgione, Marco (SUPSI)
Formentin, Simone (Politecnico Di Milano)
Bemporad, Alberto (IMT Institute for Advanced Studies Lucca) - external page Model Predictive Control Design for Dynamical Systems Learned by Echo State Networks
Bugliari Armenio, Luca (Politecnico Di Milano)
Terzi, Enrico (Politecnico Di Milano)
Farina, Marcello (Politecnico Di Milano)
Scattolini, Riccardo (Politecnico Di Milano) - external page Probabilistic Verification and Reachability Analysis of Neural Networks Via Semidefinite Programming
Faziyab, Mahyar (University of Pennsylvania)
Morari, Manfred (University of Pennsylvania)
Pappas, George J (University of Pennsylvania)
The Invited Sessions (TuB13, WeB13, ThB13 and FrB13) were very well received by CDC participants. We thank all authors for contributing to this success.
Session Overviews:
TuB13: Learning-based Control I
external page Learning Control for Polynomial Systems using Sum of Squares (1)
Guo, Meichen (University of Groningen)
De Persis, Claudio (University of Groningen)
Tesi, Pietro (University of Florence)
external page Exploiting Differential Flatness for Robust Learning-based Tracking Control Using Gaussian Processes
Greeff, Melissa (University of Toronto)
Schoellig, Angela P (University of Toronto)
external page Safe Learning-based Model Predictive Control Under State and Input-dependent Uncertainty Using Scenario Trees (1)
Bonzanini, Angelico Domenico (UC Berkeley)
Paulson, Joel (Ohio State University)
Mesbah, Ali (UC Berkeley)
external page Constrained Optimal Tracking Control of Unknown Systems: A Multi-step Linear Programming Approach (1)
Tanzanakis, Alexandros (ETH Zurich)
Lygeros, John (ETH Zurich)
WeB13: Learning-based Control 11
external page Scenario Optimization with Relaxation: A New Tool for Design and Application to Machine Learning Problems (1)
Campi, MC (Universita’ Di Brescia)
Garatti, Simone (Politecnico Di Milano)
WeB13: Learning-based Control II
external page Control Barrier Functions for Unknown Non-Linear Systems Using Gaussian Processes (1)
Jagtap, Pushpak (KTH Royal Institute of Technology)
Pappas, George J (University of Pennsylvania)
Zamani, Majid (University of Colorado, Boulder)
external page A Convex Approach to Robust LQR (1)
Scampicchio, Anna (University of Padova)
Pillonetto, Gianluigi (University of Padova)
external page Controller Design Via Experimental Exploration with Robustness Guarantees
Holicki, Tobias (University of Stuttgart)
Scherer, Carsten W (University of Stuttgart)
Trimpe, Sabastian (RWTH Aachen University)
external page Learning Control Barrier Functions from Expert Demonstrations (1)
Robey, Alexander (University of Pennsylvania)
Hu, Haimin (University of Pennsylvania)
Lindemann, Lars (Royal Institute of Technology, KTH)
Zhang, Hanwen (University of Pennsylvania)
Dimarogonas, Dimos V (KTH Royal Institute of Technology)
Tu, Stephen (University of California, Berkeley)
Matni, Nikolai (University of Pennsylvania)
external page On the Robustness of Equilibria in Generalized Aggregative Games (1)
Fabiani, Filippo (University of Oxford)
Margellos, Kostas (University of Oxford)
Goulart, Paul J (University of Oxford)
ThB13: Learning-based Control III
external page Stability and Feasibility of Neural Network-based Controllers via Output Range Analysis (I)
Karg, Benjamin (TU Berlin)
Lucia, Sergio (TU Berlin)
external page Direct Data-driven Design of Neural Reference Governors (I)
Masti, Daniele (IMT School for Advanced Studies Lucca)
Breschi, Valentina (Politecnico di Milano)
Formentin, Simone (Politecnico Di Milano)
Bemporad, Alberto (IMT School for Advanced Studies Lucca)
external page Learning Nonlinear Feedback Controllers from Data via Optimal Policy Search and Stochastic Gradient Descent (I)
Ferrarotti, Laura (IMT School for Advanced Studies Lucca)
Bemporad, Alberto (IMT School for Advanced Studies Lucca)
external page How Training Impacts Performance in Learning-based Control
Lederer, Armin (Technical University of Munich)
Capone, Alexandre (Technical University of Munich)
Umlauft, Jonas (Technical University of Munich)
Hirche, Sandra (Technische Universität München)
external page To Share or Not to Share? Performance Guarantees and the Asymmetric Nature of Cross-Robot Experience Transfer
Sorocky, Michael Joseph (University of Toronto)
Zhou, Siqi (University of Toronto)
Schoellig, Angela P (University of Toronto)
FrB13: Learning-based Control IV
external page Active Exploration in Adaptive Model Predictive Control (I)
Parsi, Anilkumar (ETH Zurich)
Ianelli, Andrea (ETH Zurich)
Smith, Roy S (ETH Zurich)
external page Computing the Racing Line Using Bayesian Optimization (I)
Jain, Achin (University of Pennsylvania)
Morari, Manfred (University of Pennsylvania)
external page Trajectory Optimization for Non-linear Multi-agent Systems Using Decentralized Learning Model Predictive Control (I)
Zhu, Edward (University of California, Berkeley)
Stürz, Yvonne R (UC Berkeley)
Rosolia, Ugo (Caltech)
Borrelli, Francesco (University of California at Berkeley)
external page Learning to Satisfy Unknown Constraints in Iterative MPC (1)
Bujarbaruah, Monimoy (UC Berkeley)
Vallon, Charlott (University of California, Berkeley)
Borelli, Francesco (University of California at Berkeley)
external page Sample Complexity of Data-driven Stochastic LQR with Multiplicative Uncertainty (I)
Coppens, Peter (KU Leuven)
Patrinos, Panagiotis (KU Leuven)
The Invited Sessions (TuA01, TuB01, WeA01 and WeB01) were very well received by CDC participants. We thank all authors for contributing to this success.
Session Overviews:
TuA01: Learning-based Control: Data-driven Methods
external page Direct Data-Driven Model-reference Control with Lyapunov Stability Guarantees (I)
Breschi, Valentina (Politecnico Di Milano)
De Persis, Claudio (University of Groningen)
Formentin, Simone (Politecnico Di Milano)
Tesi, Pietro (University of Florence)
Sabug, Lorenzo Jr. (Politecnico Di Milano)
Ruiz Fredy (Politecnico Di Milano)
Fagiano, Lorenzo (Politecnico Di Milano)
external page On the Consistency of the Risk Evaluation in the Scenario Approach (I)
Garatti, Simone (Politecnico Di Milano)
Campi, M. C. (Universita' Di Brescia)
external page System Theory without Transfer Functions and State-Space? Yes, It's Possible!
Markovsky, Ian (Vrije Universiteit Brussel)
external page From System Level Synthesis to Robust Closed-Loop Data-Enabled Predictive Control
Lian, Yingzhao (EPFL)
Jones, Colin (EPFL)
external page Data-Based System Analysis and Control of Flat Nonlinear Systems
Alsalti, Mohammen (Leibniz University Hannover)
Berberich, Julian (University of Stuttgart)
Lopez, Victor G (Leibnitz University of Hannover)
Allgöwer, Frank (University of Stuttgart)
Muller, Matthias A (Leibniz University Hannover)
TuB01: Learning-based Control: Model Predictive Control
external page Data to Controller for Nonlinear Systems: An Approximate Solution
Hendriks, Johannes (University of Newcastle)
Holdsworth, James Robert Zayas (University of Newcastle)
Wills, Adrian (University of Newcastle)
Schön, Thomas (bo) (Uppsala University)
Ninness, Brett (University of Newcastle)
external page Learning Convex Terminal Costs for Complexity Reduction in MPC (I)
Abdufattokhov, Shokhjakhon (IMT School for Advanced Studies Lucca)
Zanon, Mario (IMT School for Advanced Studies Lucca)
Bemporad, Alberto (IMT School for Advanced Studies Lucca)
external page Data-Driven Rollout for Deterministic Optimal Control (I)
Li, Yuchao (KTH Royal Institute of Technology)
Johansson, Karl H (KTH Royal Institute of Technology)
Martensson, Jonas (KTH Royal Institute of Technology)
Bertsekas, Dimitri P (MIT)
Bonzanini, Angelo Domenico (UC Berkeley)
Mesbah, Ali (UC Berkeley)
Di Cairano, Stefano (Mitsubishi Electric Research Labs)
Brunke, Lukas (University of Toronto)
Zhou, Siqi (University of Toronto)
Schoellig, Angela P (University of Toronto)
Wabersich, Kim Peter (ETH Zurich)
Krishnadas, Raamadaas (ETH Zurich)
Zeilinger, Melanie N (ETH Zurich)
WeA01: Learning-based Control: Model Learning
external page Learning Accurate Long-Term Dynamics for Model-Based Reinforcement Learning (I)
Lambert, Nathan (UC Berkeley)
Wilcox, Albert (UC Berkeley)
Zhang, Howard (UC Berkeley)
Pister, Kristofer (UC Berkeley)
Calandra, Roberto (Facebook)
external page Distributed Bayesian Online Learning for Cooperative Manipulation
Budde genannt Dohmann, Pablo (Technical University of Munich)
Lederer, Armin (Technical University of Munich)
Dissemond, Marcel (Technical University of Munich)
Hirche, Sandra (Technical University of Munich)
external page Learning ODE Models with Qualitative Structure Using Gaussian Processes (I)
Ridderbusch, Steffen (University of Oxford)
Offen, Christian (Paderborn University)
Ober-Bloebaum, Sina (University of Oxford)
Goulart, Paul J (University of Oxford)
external page Linear Systems Can Be Hard to Learn (I)
Tsiamis, Anastasios (University of Pennsylvania)
Pappas, George J (University of Pennsylvania)
external page Distributed Bayesian Parameter Inference for Physics-Informed Neural Networks
Bai, He (Oklahoma State University)
Bhar, Kinjal (Oklahoma State University)
George, Jemin (U.S. Army Research Laboratory)
Busart, Carl (U.S. Army DEVCOM ARL)
external page Structured Hammerstein-Wiener Model Learning for Model Predictive Control
Moriyasu, Ryuta (Toyota Central R&D Labs)
Ikeda, Taro (Toyota Central R&D Labs)
Kawaguchi, Sho (Toyota Industries Corporation)
Kashima, Kenji (Kyoto University)
WeB01: Learning-based Control: Controller Learning
external page Learning-Based Repetitive Precision Motion Control with Mismatch Compensation (I)
Balta, Efe C (ETH Zurich)
Barton, Kira (University of Michigan, Ann Arbor)
Tilbury, Dawn M. (University of Michigan)
Rupenyan, Alisa (ETH Zurich)
Lygeros, John (ETH Zurich)
Pauli, Patricia (University of Stuttgart)
Gramlich, Dennis (University of Stuttgart)
Barberich, Julian (University of Stuttgart)
Allgöwer, Frank (University of Stuttgart)
Yang, Fengjun (University of Pennsylvania)
Matni, Nicolai (University of Pennsylvania)
external page Inverse Reinforcement Learning: A Control Lyapunov Approach (I)
Tesfazgi, Samuel (Technical University of Munich)
Lederer, Armin (Technical University of Munich)
Hirche, Sandra (Technical University of Munich)
Paulson, Joel (The Ohio State University)
Shao, Ketong (UC Berkeley)
Mesbah, Ali (UC Berkeley)
external page Data-Driven Online Convex Optimization for Control of Dynamical Systems
Nonhoff, Marko (Leibniz University Hannover)
Muller, Matthias A (Leibniz University Hannover)
The Invited Sessions (WeAT05, WeBT05, WeCT05 and ThAT05) were very well received by CDC participants. We thank all authors for contributing to this success.
SESSION OVERVIEWS:
WeAT05 - Learning-Based Control I: Data-Driven Predictive Control
external page Data-Enabled Predictive Control with Instrumental Variables: The Direct Equivalence with Subspace Predictive Control
van Wingerden, Jan-Willem
Mulders, Sebastiaan P.
Dinkla, Rogier
Oomen, Tom
Verhaegen, Michel
external page Data-Driven Multiple Shooting for Stochastic Optimal Control
Ou, Ruchuan
Pan, Guanru
Faulwasser, Timm
external page An Offset-Free Nonlinear MPC Scheme for Systems Learned by Neural NARX Models
Bonassi, Fabio
Xie, Jing
Farina, Marcello
Scattolini, Riccardo
external page Data-Driven Nonlinear Model Reduction Using Koopman Theory: Integrated Control Form and NMPC Case Study
Schulze, Jan C.
Mitsos, Alexander
external page Compression at the Service of Learning: A Case Study for the Guaranteed Error Machine
Garatti, Simone
C. Campi, Marco
external page Learning Functions and Uncertainty Sets Using Geometrically Constrained Kernel Regression
Fiedler, Christian
Scherer, Carsten W.
Trimpe, Sebastian
WeBT05 - Learning-Based Control II: Analysis, Estimation and Control
external page On a Continuous-Time Version of Willems' Lemma
Lopez, Victor G.
Müller, Matthias A.
external page Neural System Level Synthesis: Learning Over All Stabilizing Policies for Nonlinear Systems
Furieri, Luca
Galimberti, Clara Lucía
Ferrari-Trecate, Giancarlo
external page Data-Driven Input Reconstruction and Experimental Validation
Shi, Jicheng
Lian, Yingzhao
Jones, Colin N.
external page Linear Observer Learning by Temporal Difference
Menchetti, Stefano
Zanon, Mario
Bemporad, Alberto
external page Data-Driven Analysis and Design Beyond Common Lyapunov Functions
van Waarde, Henk J.
Camlibel, M. Kanat
Trentelman, Harry L.
external page Finite-Sample Guarantees for State-Space System Identification under Full State Measurements
Baggio, Giacomo
Carè, Algo
Pillonetto, Gianluigi
WeCT05 - Learning-Based Control III: Robustness and Safety
external page A System Level Approach to Regret Optimal Control
Didier, Alexandre
Sieber, Jerome
Zeilinger, Melanie N.
external page Improved Rates for Derivative Free Gradient Play in Strongly Monotone Games
Drusvyatskiy, Dmitriy
Fazel, Maryam
Ratliff, Lillian J.
external page Safe Reinforcement Learning Via Confidence-Based Filters
Curi, Sebastian
Lederer, Armin
Hirche, Sandra
Krause, Andreas
external page Performance-Robustness Tradeoffs in Adversarially Robust Linear-Quadratic Control
Lee, Bruce D.
Zhang, Thomas T.C.K.
Hassani, Hamed
Matni, Nikolai
external page Improving the Performance of Robust Control through Event-Triggered Learning
von Rohr, Alexander
Solowjow, Friedrich
Trimpe, Sebastian
external page Scalable Estimation of Invariant Sets for Mixed-Integer Nonlinear Systems Using Active Deep Learning
Bonzanini, Angelo D.
Paulson, Joel A.
Makrygiorgos, Georgios
Mesbah, Ali
ThAT05 - Learning-Based Control IV: Controller Design and Applications
external page On Controller Tuning with Time-Varying Bayesian Optimization
Brunzema, Paul
von Rohr, Alexander
Trimpe, Sebastian
external page Neural Energy Casimir Control for Port-Hamiltonian Systems
Xu, Liang
Zakwan, Muhammad
Ferrari-Trecate, Giancarlo
external page Structured-Policy Q-Learning: An LMI-Based Design Strategy for Distributed Reinforcement Learning
Sforni, Lorenzo
Camisa, Andrea
Notarstefano, Giuseppe
external page Convex Analytic Theory for Convex Q-Learning
Lu, Fan
Mehta, Prashant G.
Meyn, Sean P.
Neu, Gergely
external page Multi-Agent Deep Reinforcement Learning for Shock Wave Detection and Dissipation Using Vehicle-To-Vehicle Communication
Suriyarachchi, Nilesh
Noorani, Erfaun
Tariq, Faizan M.
Baras, John S.
external page Physically Consistent Learning of Conservative Lagrangian Systems with Gaussian Processes
Evangelisti, Giulio
Hirche, Sandra
The Invited Sessions (WeA02, WeB02, ThA02 and ThB02) were very well received by CDC participants. We thank all authors for contributing to this success.
SESSION OVERVIEWS:
WeA02: Learning-Based Control I: Policy Learning and Optimization
external page Differentiable Sparse Optimal Control
Shima, Ryotaro (Toyota Central R&D Labs)
Moriyasu, Ryuta (Toyota Central R&D Labs)
Kawaguchi, Sho (Toyota Industries Corporation)
Kashima, Kenji (Kyoto University)
external page An Efficient Off-Policy Reinforcement Learning Algorithm for the Continuous-Time LQR Problem (I)
Lopez, Victor G. (Leibniz University Hannover)
Müller, Matthias A. (Leibniz University Hannover)
external page No-Regret Bayesian Optimization with Gradients Using Local Optimality-Based Constraints: Application to Closed-Loop Policy Search (I)
Makrygiorgos, Georgios (University of California, Berkeley)
Paulson, Joel (The Ohio State University)
Mesbah, Ali (University of California, Berkeley)
external page Scenario Optimization with Constraint Relaxation in a Non-Convex Setup: A Flexible and General Framework for Data-Driven Design (I)
Garatti, Simone (Politecnico Di Milano)
Campi, M. C. (Universita' Di Brescia)
external page On-Policy Data-Driven Linear Quadratic Regulator Via Model Reference Adaptive Reinforcement Learning (I)
Borghesi, Marco (University of Bologna)
Bosso, Alessandro (University of Bologna)
Notarstefano, Giuseppe (University of Bologna)
external page Learning Optimal Policies in Mean Field Models with Kullback-Leibler Regularization (I)
Busic, Ana (Inria)
Meyn, Sean P. (Univ. of Florida)
Cammardella, Neil (University of Florida)
WeB02: Learning-Based Control II: Safety and Robustness
external page A Computationally Lightweight Safe Learning Algorithm (I)
Baumann, Dominik (Aalto University)
Kowalczyk, Krzysztof (Wroclaw University of Science and Technology)
Tiels, Koen (Eindhoven University of Technology)
Wachel, PawelWroclaw (University of Science and Technology, Poland)
external page Learning Over Contracting and Lipschitz Closed-Loops for Partially-Observed Nonlinear Systems (I)
Barbara, Nicholas H. (The University of Sydney
Wang, Ruigang (The University of Sydney)
Manchester, Ian R. (University of Sydney)
external page An Online Learning Analysis of Minimax Adaptive Control (I)
Renganathan, Venkatraman (Lund University)
Iannelli, Andrea (University of Stuttgart)
Rantzer, Anders (Lund University)
external page Risk-Sensitive Inhibitory Control for Safe Reinforcement Learning (I)
Lederer, Armin (Technical University of Munich)
Noorani, Erfaun (University of Maryland College Park)
Baras, John S. (University of Maryland)
Hirche, Sandra (Technische Universität München)
external page Learning Safety Filters for Unknown Discrete-Time Linear Systems
Farokhi, Farhad (The University of Melbourne)
Leong, Alex S. (DST Group)
Zamani, Mohammad (University of Melbourne)
Shames, Iman (Australian National University)
external page Data-Driven Synthesis of Safety Controllers for Partially-Observable Systems with Unknown Models (I)
Jahanshahi, Niloofar (Ludwig Maximilian University of Munich)
Zamani, Majid (University of Colorado Boulder)
ThA02: Learning-Based Control III: Model Learning, Analysis and Control
external page Physically Consistent Multiple-Step Data-Driven Predictions Using Physics-Based Filters
Lian, YingzhaoEPFLShi, JichengEPFLJones, Colin N.EPFL 10:20-10:40, Paper ThA02.2 Add to
external page Data-Driven Feedback Linearization with Complete Dictionaries (I)
De Persis, Claudio (University of Groningen)
Gadginmath, Darshan (University of California, Riverside)
Pasqualetti, Fabio (University of California, Riverside)
Tesi, Pietro (Università Degli Studi Di Firenze)
external page Unconstrained Parametrization of Dissipative and Contracting Neural Ordinary Differential Equations (I)
Martinelli, Daniele (EPFL)
Galimberti, Clara Lucía (EPFL)
Manchester, Ian R. (University of Sydney)
Furieri, Luca (EPFL)
Ferrari-Trecate, Giancarlo (EPFL)
external page Abstracting Linear Stochastic Systems Via Knowledge Filtering (I)
Engelaar, Maico Hendrikus Wilhelmus (Eindhoven University of Technology)
Romao, Licio (University of Oxford)
Gao, Yulong (University of Oxford)
Lazar, Mircea (Eindhoven University of Technology)
Abate, Alessandro (University of Oxford)
Haesaert, Sofie (Eindhoven University of Technology)
external page Learning Control of Second-Order Systems Via Nonlinearity Cancellation (I)
Guo, Meichen (Delft University of Technology)
De Persis, Claudio (University of Groningen)
Tesi, Pietro (University of Florence)
external page On the Impact of Regularization in Data-Driven Predictive Control (I)
Breschi, Valentina (Eindhoven University of Technology)
Chiuso, Alessandro (University of Padua)
Fabris, Marco (University of Padua)
Formentin, Simone (Politecnico Di Milano)
ThB02: Learning-Based Control IV: Data-Driven Controller Design
external page Data-Driven Model-Reference Control with Closed-Loop Stability: The Output-Feedback Case
de Jong, Thomas O. (Eindhoven University of Technology)
Breschi, Valentina (Eindhoven University of Technology)
Schoukens, Maarten (Eindhoven University of Technology)
Formentin, Simone (Politecnico Di Milano)
external page Model-Free Data-Driven Predictive Control Using Reinforcement Learning (I)
Sawant, Shambhuraj (NTNU Trondheim)
Reinhardt, Dirk Peter (Norwegian University of Science and Technology)
Bahari Kordabad, Arash (Norwegian University of Science and Technology)
Gros, Sebastien (NTNU)
external page The Fundamental Limitations of Learning Linear-Quadratic Regulators (I)
Lee, Bruce (University of Pennsylvania)
Ziemann, Ingvar (University of Pennsylvania)
Tsiamis, Anastasios (ETH Zurich)
Sandberg, Henrik (KTH Royal Institute of Technology)
Matni, Nikolai (University of Pennsylvania)
external page On the Design of Persistently Exciting Inputs for Data-Driven Control of Linear and Nonlinear Systems
Alsalti, Mohammad (Leibniz University Hannover)
Lopez, Victor G. (Leibniz University Hannover)
Müller, Matthias A. (Leibniz University Hannover)
external page Online Control for Adaptive Tapering of Medications (I)
Gradu, Paula (University of California, Berkeley)
Recht, Benjamin (University of California, Berkeley)
external page Differentially Flat Learning-Based Model Predictive Control Using a Stability, State, and Input Constraining Safety Filter
Hall, Adam W. (University of Toronto)
Greeff, Melissa (University of Toronto)
Schoellig, Angela P. (University of Toronto)